3.12 Data-Management Protocol
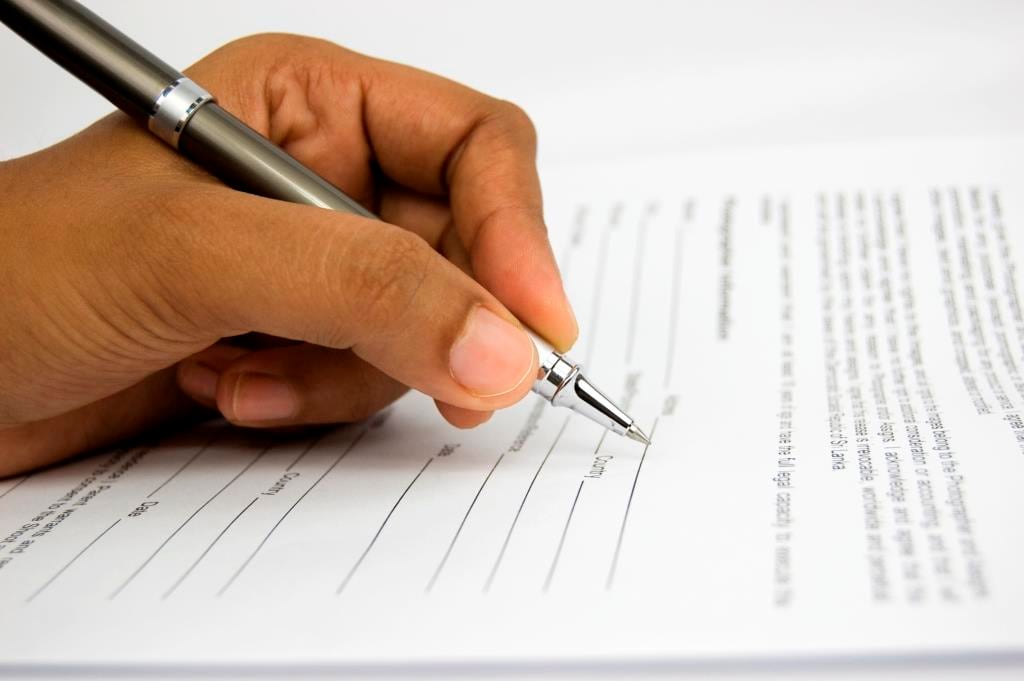
On this Page
Possible responses:
- Improves data quality through standardization of data management
- Creates procedural transparency
- Improves data transmission
- Ensures data confidentiality and security
- Defines the roles of data management in each step
Activity 3.5
Ask participants to refer to Activity 3.5 in the Participant Workbook pdf icon[457 KB, 21 Pages, Print Only].
After participants complete their flow chart, show the following chart with possible responses and discuss participants’ responses.
It is important that all participating personnel are trained in the data-management protocol. The protocol for data collection and management should include procedures for:
- Identification of data sources where congenital anomalies can be identified
- Identification and registration of congenital anomalies by health-care professionals in each data source
- Training of personnel responsible for coding congenital anomalies according to the ICD-10 coding system
- Taking photographs of a fetus or neonate with congenital anomalies, if appropriate for the setting
- Verifying the information at the participating hospital site
- Sending information to a regional or national-level surveillance programme
It is important that the data collection and analysis are done in a systematic way by trained personnel, to ensure high-quality data and accurate interpretation. Poor-quality data can lead to false conclusions about the occurrence of a congenital anomaly in a population, and weaken the credibility of the programme. Inaccurate interpretation could have a substantial impact on the decisionmaking process of public health authorities.
Attributes
Possible responses:
- Completeness
- Accuracy
- Timeliness
Completeness refers to the extent to which data are comprehensive. For example, all cases at a given source in a specific timeframe have been identified, and all required data have been abstracted. Hospital audits and linkage of cases to other sources of data, such as vital records or specialized diagnostic centres, can help evaluate the completeness of case ascertainment.
Accuracy refers to the extent to which data are exact, correct and valid. Approaches to help ensure data accuracy include:
- Re-abstraction of information
- Validity audits, such as identification of missed diagnoses or coding issues
- Clinical reviews, such as verification of diagnoses, codes assigned, tests and procedures (i.e. two separate individuals input the same data and see if the database identified the duplicate)
- Verification of data entry, such as customized programmes for range checks, automated fields, rejection of data that are known to be inaccurate, and routinely running data queries to identify duplicate entries and problems with variables
Timeliness refers to the extent to which data are collected and analysed in a timely manner. It is measured by the time that elapses between the date of diagnosis and the date of abstraction; the date of abstraction and the date information is sent to the office; the date of arrival in the office to the date it is entered in the system; and the date of final product development and dissemination.
Accuracy and timeliness also refer to how the data are reported to target audiences.
Factors Affecting Data Quality
Possible responses:
- Missing values or empty data fields in the abstraction form
- Duplication of cases
- Errors in the diagnosis, description and/or coding of congenital anomalies