Looking at AI’s Potential Impact on Health Equity
As new technology reveals what couldn’t be seen before, we’re also looking at its future impacts on public health
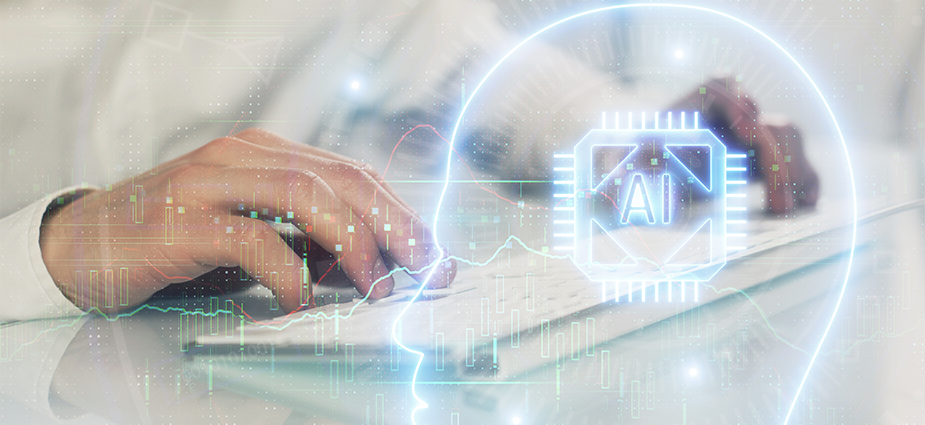
CDC is exploring new applications of artificial intelligence and machine learning (AI/ML) for public health, such as forecasting trends in opioid overdose mortality, using natural language processing methods on foodborne outbreak data, and using large language models to improve syndromic surveillance.
These cutting-edge technologies offer huge benefits for public health. They can help process massive amounts of data that are hard for humans to do at scale. We can also discover relationships in the data that are hard for traditional methods to find.
As we incorporate more artificial intelligence into our data workflows, we also need to look at how to prevent potential biases in the data that may impact health equity.
Understanding biases
The first step is to recognize that our current data and methods may already contain different types of biases. For example, missing information — such as when data on race, ethnicity, or social determinants of health aren’t being fully collected — can create biases in the underlying data. This can result in people being under-represented in the data and underserved by public health.
Biases can also arise from how data are being analyzed or interpreted. If we apply new technologies like artificial intelligence without considering these issues, the risk is that we could make the problem larger instead of solving it.
Developing a framework
Taking the right measures early on can help us identify and prevent biases at every step. But what are the right measures we should be taking?
To answer that question, CDC’s Office of Science teamed up with experts from Georgia Tech Research Institute. Together, we’re working to develop guidance and training resources that will help public health researchers effectively navigate many of the health equity-related challenges they encounter when conducting data science using health data.
The goal is to find the right tools and techniques to consider and assess health equity from end-to-end. This means looking at every stage of the workflow – the data sources themselves, how data are prepared, and how they are analyzed and reported.
Moving toward equity
As we gain more understanding of the scope of AI’s impact on our public health processes, we will uncover which improvements will give us the greatest potential to increase health equity. We’re aiming to get a holistic view of what our practices look like around these new technologies, so that we can achieve more equitable health outcomes.
This work is an important part of executing CDC’s strategies for data modernization and health equity and recognizing that this work must go hand-in-hand if we are to truly serve everyone in America.